2024 Graduate Studentship Award Recipients

The Institute for Pandemics (IfP) is pleased to announce the seven recipients of the 2024 Graduate Studentship Awards.
The purpose of the $10,000 awards is to foster interdisciplinary research training in pandemic readiness, resilience and/or recovery. All awardees work on projects that are co-supervised by faculties from different disciplines. The studentship program provides the opportunity to participate in IfP programming and activities, attend workshops and seminars, present their research at the IfP Symposium, and network with IfP members across divisions and campuses at the University of Toronto.
List of the Graduate Studentship awardees
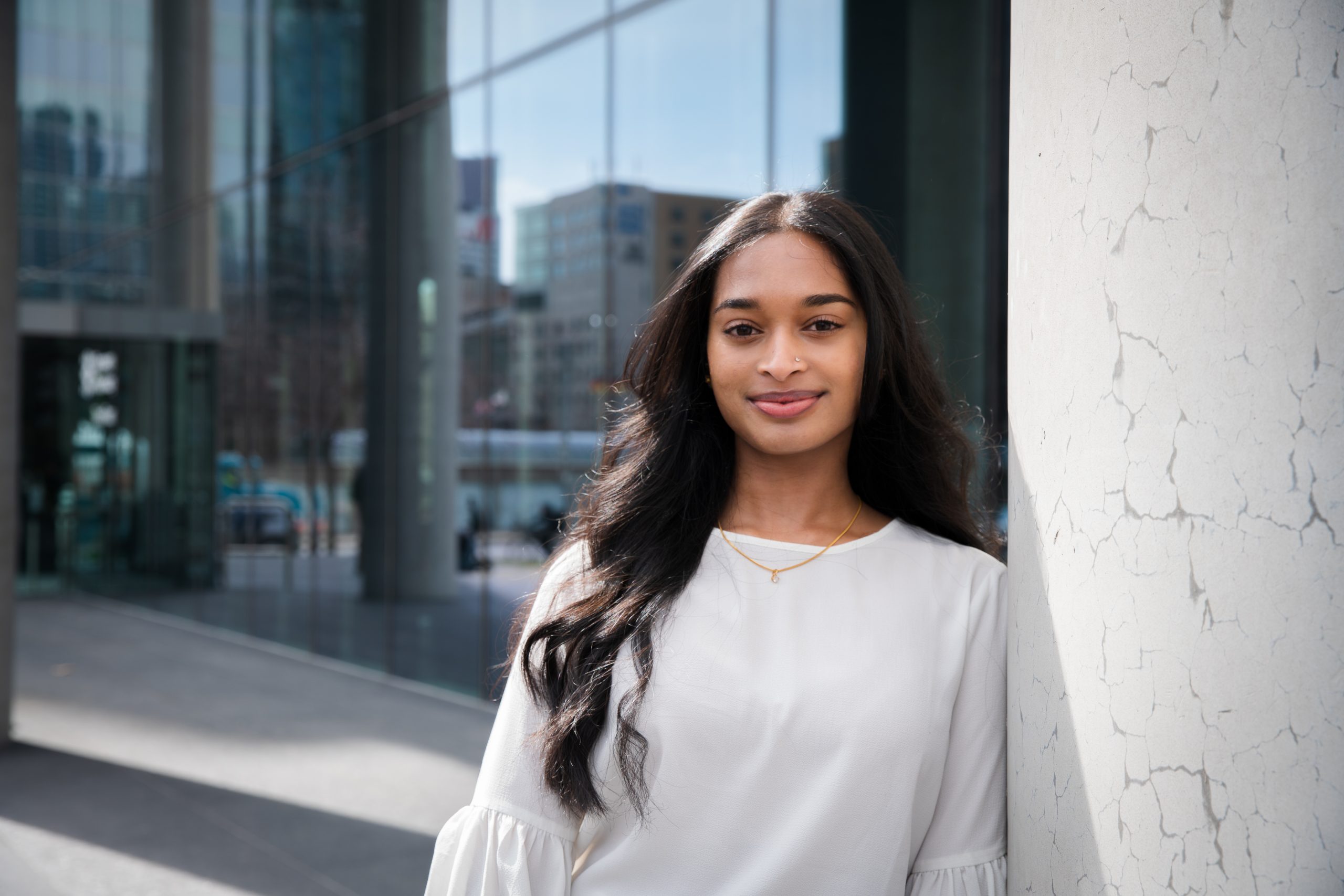
Supervised by Mina Tadrous, Leslie Dan Faculty of Pharmacy and Étienne Gaudette, Dalla Lana School of Public Health
Project Title: Impact of Canadian drug shortages on drug utilization and clinical outcomes
Project Summary: Drug shortages are a growing concern in Canada. Over 13,000 drugs were at risk for shortage over the last five years, with almost half facing at least one shortage. Drug shortages are a multifaceted global problem which can be attributed to the complex multinational drug supply chain. Weaknesses of this drug supply chain have been highlighted during the recent COVID-19 pandemic, seen as supply chain disruptions and inequitable allocation of treatments. Poor pandemic readiness and resilience led to increased shortages and impaired patient access to medicines. However, two years after the pandemic, shortages continue to impact the healthcare system and the quality of care delivered. The impact of shortages on drug utilization trends is understudied, making it difficult to comprehend the manifestation of shortages at the patient level. Our project aims to 1) understand the impact of drug supply disruptions on drug utilization reported in observational studies, 2) describe drug utilization trends in response to Canadian shortages and develop a predictive model to anticipate shortage-risk, 3) Investigate the impact of the ranitidine recall on the delivery of treatment and clinical outcomes. These finding will generate the necessary real-world evidence to guide policy decisions on shortage mitigation strategies ensuring pandemic preparedness, and resilience.
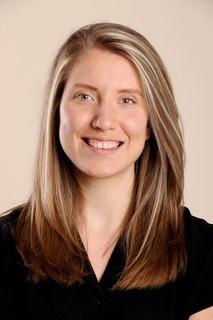
Supervised by Patrick Brown, Faculty of Arts & Science, Monica Alexander, Faculty of Arts & Science, and Lennon Li, Dalla Lana School of Public Health
Project Title: A spatio-temporal framework for estimation and interpretation of wastewater viral signals
Project Summary: Wastewater-based surveillance stands as a forefront method for disease monitoring, offering a real-time, representative depiction of disease presence, irrespective of access to clinical testing or symptom status. Currently, a wide range of pathogens, including SARS-CoV-2, influenza, hepatitis, and respiratory syncytial virus (RSV), are being monitored through wastewater, highlighting its potential for proactive public health management.
Public health researchers want reliable estimates of the viral signal and its direction, for public dissemination. Wastewater modeling presents challenges due to low signal-to-noise ratios, unequal sampling, temporal correlation, and signal variability across communities. Improved statistical methods are needed to separate signal from noise and articulate uncertainty in wastewater trends. Simultaneously, to ensure effective public dissemination, these advanced models need to run in a timely manner.
We propose a flexible, fast, Bayesian hierarchical modeling framework adaptable to all pathogens, sampling schedules and availability of control measurements for normalization. Moreover, we propose modelling viral signals as differentiable processes, enabling direct estimation of their derivatives. This way, viral signals can be conveniently presented alongside their derivatives, with uncertainty intervals, as instantaneous indicators of change. We will share our established models with the community through an open-source, user-friendly R package.
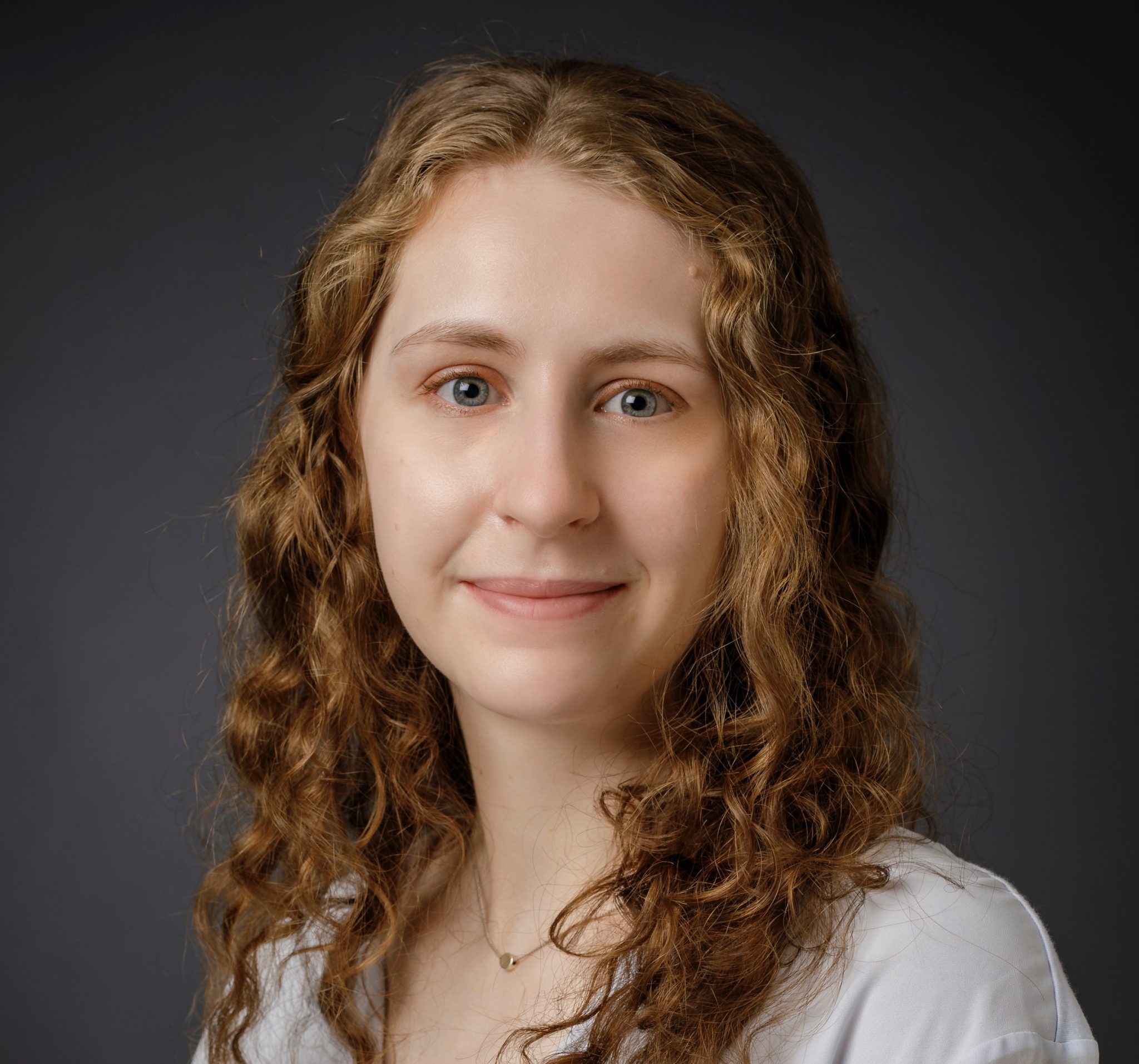
Supervised by Samira Mubareka, Temerty Faculty of Medicine and Nicole Mideo, Faculty of Applied Science & Engineering
Project Title: Genomic Investigation of Avian Influenza Viruses
Project Summary: Avian influenza viruses (AIVs) are one of the leading threats to future pandemic emergence, and have directly led to many pandemics throughout human history. My project takes advantage of high-throughput sequencing techniques to investigate the genetics of AIVs in multiple contexts. The first is surveillance, which is essential for adequate pandemic preparedness since researchers, clinicians, and public health officials can know what potential pandemic viruses look like and be specific in their preparation efforts. I am working with collaborators to sample wild and domestic animals in Ontario likely to be infected with AIVs and will use sequencing to obtain genetic information on the AIVs present in Ontario. The second aim of my project will inform AIV vaccine production methods, which are one of the most important aspects of pandemic preparedness and response. Like all influenza viruses, AIVs obtain genetic changes, called mutations, as they replicate. Current influenza virus vaccine production methods involve replicating large amounts of viruses, potentially leading to many mutations which could impact immune responses to those vaccines. My project involves replicating AIVs with the same methods used in vaccine production and then using sequencing to observe how the viruses are evolving genetically.
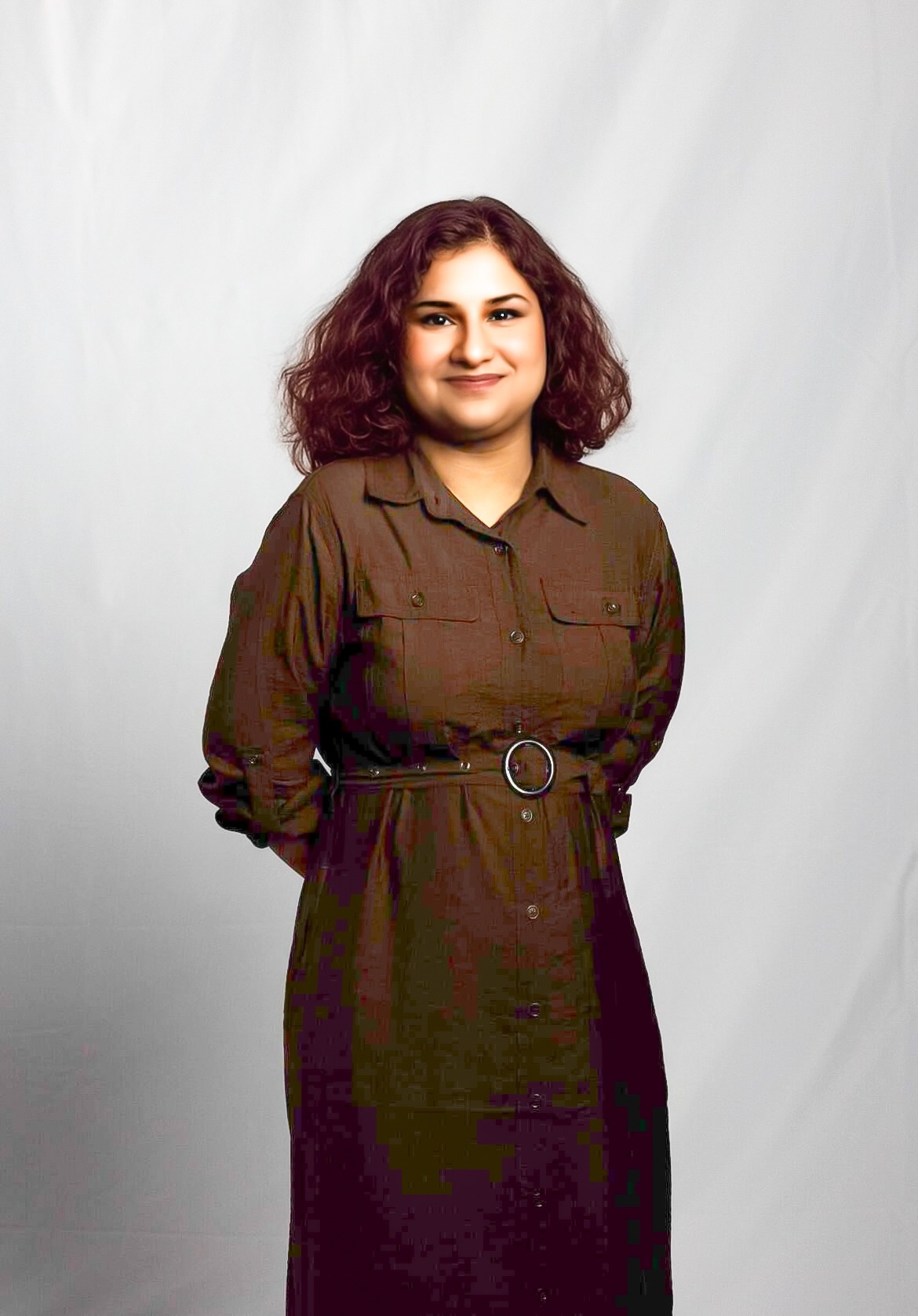
Supervised by Michael Liut, Faculty of Arts & Science, Tingting Zhu, University of Toronto Mississauga, and Carolina Nobre, Faculty of Arts & Science
Project Title: Scaling Self-Explanations in Online Learning Environment Systems
Project Summary: The pandemic-induced transition to online learning platforms revealed a trend among students towards superficial learning methods, such as accelerated video playback and haphazard answer attempts, often accompanied by environmental distractions. This project aims to bolster pandemic readiness and educational resilience by fostering deep learning through large language model (LLM)-enhanced self-explanation techniques. Recognizing the barriers of language proficiency and cognitive overload, our approach leverages voice input and intelligent prompts to facilitate self-explanation, enabling students to articulate concepts in their own words. This process not only curbs the tendency to game educational systems but also supports students in organizing thoughts coherently, irrespective of their native language. The integration of LLMs provides adaptive feedback, simulating the critical presence of educators in virtual environments. Given the need for online videos and lectures again in the event of a pandemic, such systems can help encourage learning and provide students with feedback even when they are not sitting in front of educators in class.
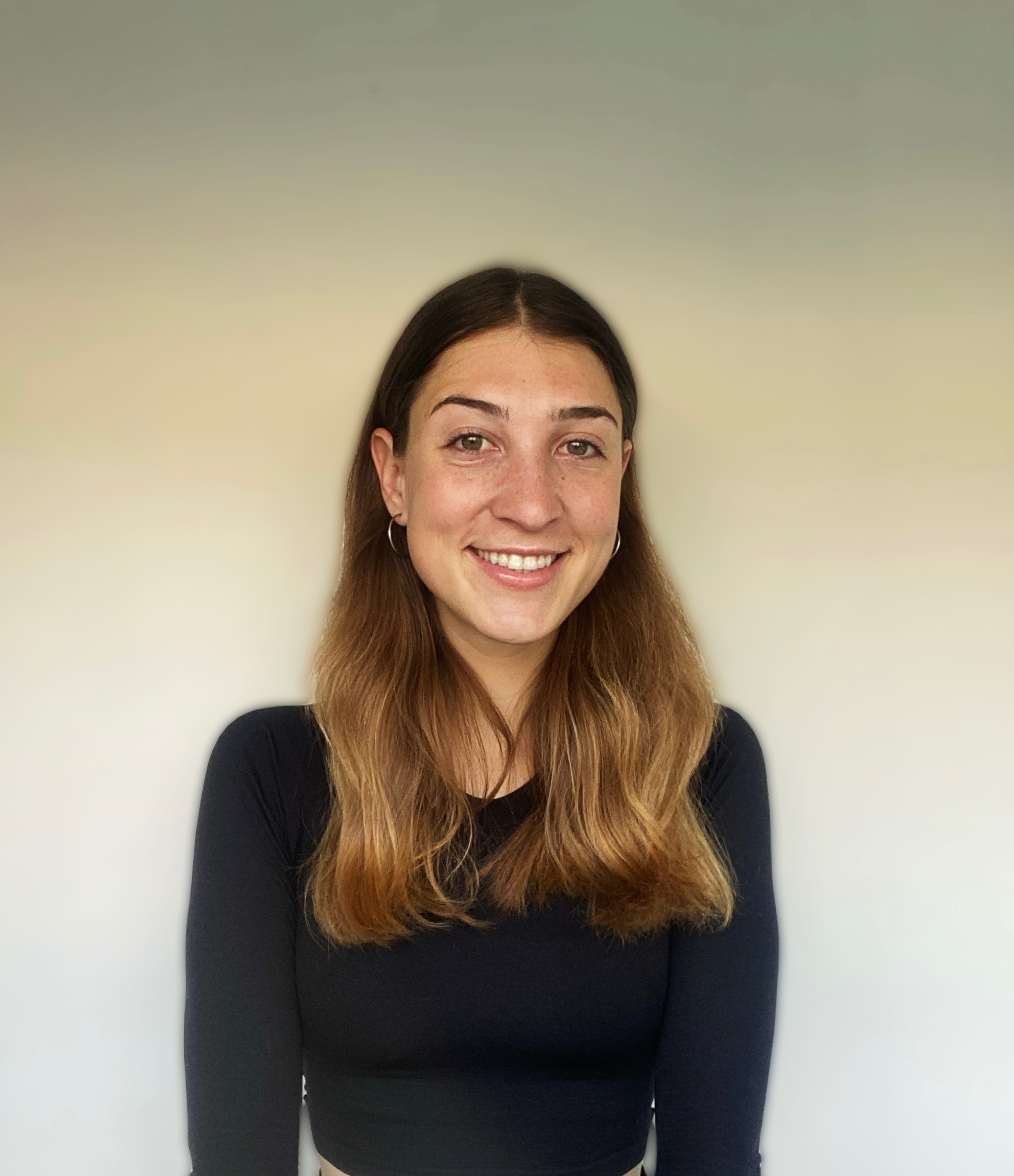
Supervised by Landon J. Edgar, Temerty Faculty of Medicine and Helen Tran, Faculty of Arts & Science
Project Title: Biosensing of Influenza A using carbohydrate-based polymers
Project Summary: One billion people become infected with the respiratory infection Influenza, annually. Influenza A (IAV) is the most prevalent influenza virus and upon a jump from avian species to humans caused four global pandemics to date. Determining whether a variant can undergo human transmission is an essential method for pandemic preparedness. Unfortunately, standard PCR testing can only detect the presence of a virus, not its potential for human transmission. I will overcome this diagnostic limitation by fabricating a biosensor that can selectively detect IAVs capable of human transmission, thereby identifying pandemic concerns earlier than present methods. Surface receptors on avian and human IAVs have distinct biological targets. In the human epithelial airway, the target is a cell-surface carbohydrate that human IAVs bind to cause infection. Using chemical approaches, I synthesized a biopolymer analogous to this carbohydrate for implementation into an electronic sensor. When a human IAV encounters and binds the biopolymer, its negatively charged shell elicits a repulsive force against the device interface that can measured. These electronic sensors are designed to be highly sensitive, and able to detect sample binding events at extremely low concentrations. This technology will be applied to test emerging IAVs for their ability to infect humans and cause pandemics.

Supervised by Zahra Shakeri, Dalla Lana School of Public Health, Aya Mitani, Dalla Lana School of Public Health, and Kuan Liu, Dalla Lana School of Public Health
Project Title: A causal inference approach to equitably characterize long COVID across geographic clusters
Project Summary:
Long COVID is a global health crisis inadequately addressed by current medical and public health measures. However, the causal risk factors of PCC for different individuals across Canada’s multicentre healthcare system are still unclear. As such, this project aims to quantify the impact of health disparities on PCC across Canada’s diverse subpopulations. To do so, we propose an innovative algorithm which combines causal inference, survival analysis, and clustered data analysis. Although these concepts and their pairwise combinations have been thoroughly researched and validated in isolation, we are amongst the first to explore all three together. To provide a brief technical outline, our proposed method extends inverse probability of treatment and censoring (IPTC) weighting, a method used in causal survival analysis, to two different clustering perspectives: central clustering and incidental clustering.
Our project consists of three primary components: the development and programming of our proposed algorithm, a simulation study of our causal survival analysis methods under varying multi-level environments and clustering perspectives, and a tutorial paper to assist with the knowledge translation of our new approach for non-technical audiences. All our R code will be shared on GitHub to ensure reproducibility and transparency, and we also hope to develop an R package for our algorithm if time permits.
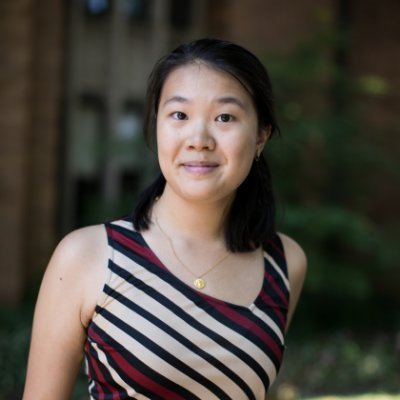
Supervised by James Scott, Dalla Lana School of Public Health, Tracy Kirkham, Ontario Health, and Alison McGeer, Temerty Faculty of Medicine
Project Title: Validation of Respirator Fit on Ontario Paramedics and Firefighters
Project Summary: During the COVID-19 pandemic, emergency service workers (ESWs) relied heavily on respirators as protection against infectious bioaerosols. However, respirators may fail when ESWs perform more physically demanding tasks (e.g., cardiopulmonary resuscitation), putting their health at risk.
I am conducting an experimental study of 200 Ontario ESWs (paramedics and firefighters) to investigate respirator fit during simulated work tasks and for respirator fit factors. Investigation into fit factors will include facial and physiological measurements, and behaviours during tasks, with data partitioned based on sex and ethnicity. This study will help elucidate whether emergency service workers are protected by respirators while performing these tasks.
Finding that tasks or other variables lead to respirators failing that previously passed standard fit tests would support changes in respiratory use among paramedics and/or changes to fit testing procedures. Such changes may reduce occupational respiratory disease in paramedics and will help inform respirator fit testing for paramedics, firefighters and allied healthcare workers in preparation for future pandemics. Other quantitative measures such as facial measurements will be linked to respirator fit to elucidate possible links with facial dimensions and respirator fit for both simulated work and standard fit tests. This measurement will help inform the potential development of new respirator designs.